Xuexin Wei
- Assistant Professor
- Neuroscience
Accepting graduate students 25-26 academic year
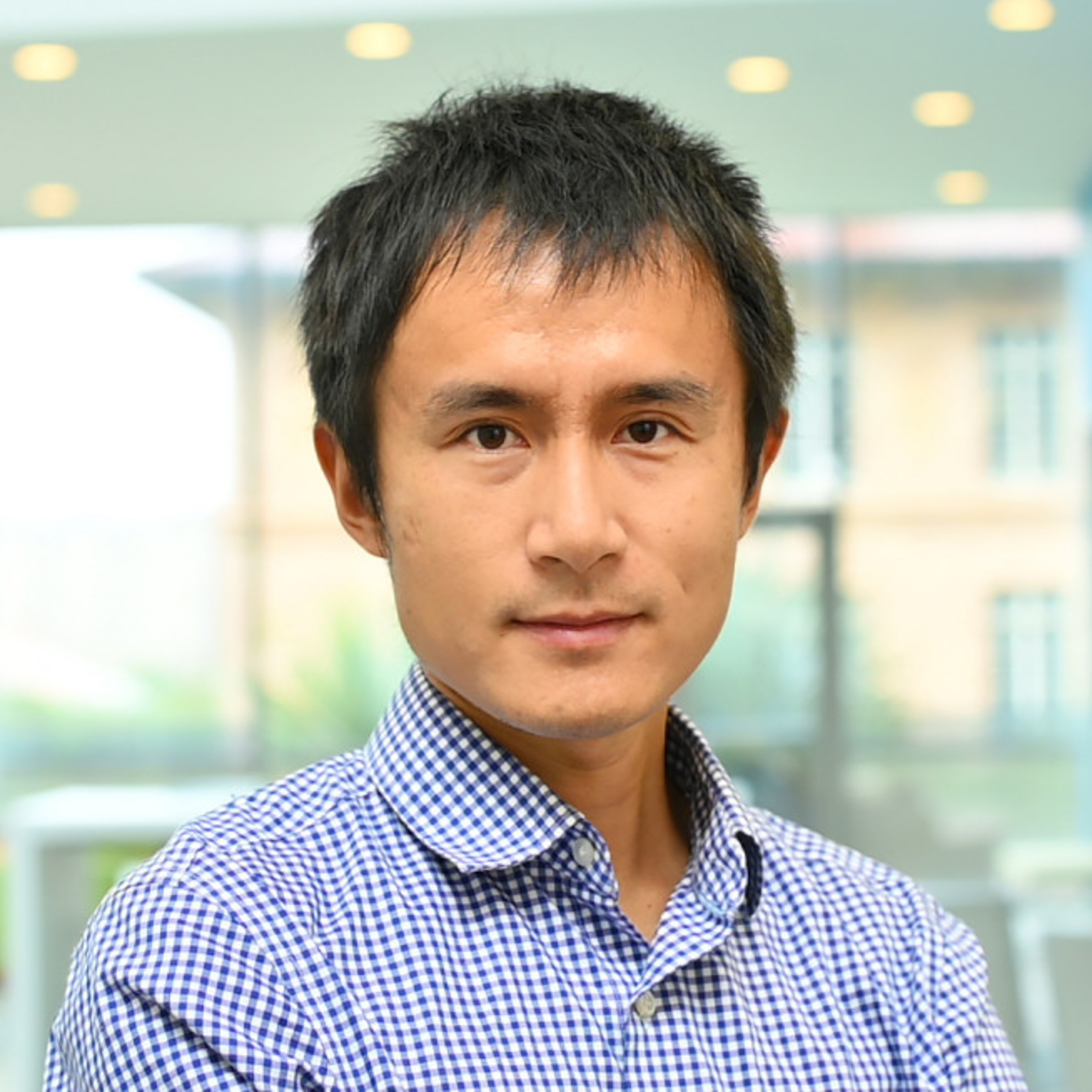
Contact Information
Biography
Xue-Xin Wei grew up in Qingdao (or Tsingtao), China. After obtaining a Bachelor degree in mathematics and applied mathematics at Peking University, he became fascinated by the inner workings of the brain. In 2010 he moved to UPenn to pursue a Ph.D in psychology. He was the recipient of Louis B. Flexner Award, which gives to the Outstanding Thesis Work on any topics related to Neurosciences at UPenn. Since 2016, he has been working as a postdoctoral research scientist at Center for Theoretical Neuroscience & Department of Statistics at Columbia University. In January 2020, Xue-Xin started as an Assistant professor in Department of Neuroscience.
Dr. Wei can be reached at weixx@utexas.edu.
Research
The lab aims to understand how brain/neurons generate adaptive and intelligent behavior. To tackle this fundamental question, we take a combination of bottom-up (analyzing experimental data and construct constrained mechanistic models ) and top-down (normative models) approach. Our research covers a variety of topics, including 1) using efficient computation as a guiding principle to understand cognition; 2) sensory adaptation; 3) normative models of visual physiology and perception; 4) understanding neural coding through geometry; 5) generalization in the brain and artificial intelligence systems; 6) building probabilistic models of neural population responses. Much of the research is in the domains of vision and navigation.
The lab works at the intersection of Computational Neuroscience, Statistics, and AI/deep learning. We leverage the computational power of deep learning to help understand computations in the brain. We are also interested in using the prior knowledge and constraints obtained from the brain to develop more powerful AI systems. We work closely with experimentalists to develop sophisticated statistical techniques to understand how the neural responses are determined by the inputs and internal states on a trial-by-trial basis. These statistical models could provide specific constraints on the operations at the neural circuit level. As a further step, we can construct quantitative models constrained by these observations to reveal computational mechanisms underlying neural computation.
We are looking for motivated graduate students, undergraduate students and postdocs to work on these problems together. Currently, there are multiple openings in the lab. Please get in touch if you are interested.
Dr. Wei can be reached at weixx@utexas.edu.
Research Areas
- Artificial Intelligence and/or Robotics
- AI for Health or Computational Science
- Statistics, Big Data or Machine Learning
- Neuroscience
Fields of Interest
- Computational
- Cognition/Sensory Systems
- Learning/Memory/Plasticity
- Computational/Theoretical
Publications
16. Deng, X., Chen, S., Sosa, M., Karlsson, M. P., Wei, X. X., & Frank, L. M. (2022). A Variable Clock Underlies Internally Generated Hippocampal Sequences. Journal of Neuroscience. Accepted.
15. Ajabi, Z., Keinath, A.T., Wei, X.X., Brandon, M.P. (2021). Population dynamics of the thalamic head direction system during drift and reorientation. bioRxiv preprint
14. Kriegeskorte, N.*, & Wei, X. X.* (2021). Neural tuning and representational geometry. Nature Reviews Neuroscience.
13. Gao, R., Xie, J., Wei, X.X., Zhu, S.C., Wu, Y.N. (2021). On Path Integration of Grid Cells: Group Representation and Isotropic Scaling, In Advances in neural information processing systems (NeurIPS).
12. Zhou, D. & Wei, X. X. (2020) Learning identifiable and interpretable latent models of high-dimensional neural activity using pi-VAE. In Advances in neural information processing systems (NeurIPS).
11. Cueva, C. J.*, Wang, P. Y.*, Chin, M.*, & Wei, X. X. (2020). Emergence of functional and structural properties of the head direction system by optimization of recurrent neural networks. International Conference on Learning Representations (ICLR, spotlight presentation).
10. Wei, X. X.*, Zhou, D.*, Grosmark, A., Ajabi, Z., Sparks, F., Zhou, P., Brandon, M., Losonczy, A., & Paninski, L. (2020). A zero-inflated gamma model for post-deconvolved calcium imaging traces. Published in Neurons, Behavior, Data analysis, and Theory (the NBDT journal).
9. Zhang, R.Y., Wei, X.X., and Kay, K. (2020) Understanding multivariate brain activity: evaluating the effect of voxelwise noise correlations on population codes in functional magnetic resonance imaging. PLOS Computational Biology 16.8: e1008153.
8. Cueva, C. J., & Wei, X. X. (2018). Emergence of grid-like representations by training recurrent neural networks to perform spatial localization. International Conference on Learning Representations (ICLR).
7. Pollock, E., Desai, N., Wei, X.X.*, and Vijay Balasubramanian, V.*. (2018) Dynamic self-organized error-correction of grid cells by border cells. arXiv preprint, arXiv:1808.0150.
6. Wei, X. X., & Stocker, A. A. (2017). Lawful relation between perceptual bias and discriminability. Proceedings of the National Academy of Sciences, 114(38), 10244-10249.
5. Wei, X. X., & Stocker, A. A. (2016). Mutual information, Fisher information, and efficient coding. Neural Computation, 28(2), 305-326.
4. Wang, Z.*, Wei, X. X*., Stocker, A. A., & Lee, D. D. (2016). Efficient neural codes under metabolic constraints. In Advances in neural information processing systems (NeurIPS, pp. 4619-4627).
3. Wei, X. X., & Stocker, A. A. (2015). A Bayesian observer model constrained by efficient coding can explain ‘anti-Bayesian' percepts. Nature Neuroscience, 18(10), 1509.
2. Wei, X. X., Prentice, J., & Balasubramanian, V.B. (2015). A principle of economy predicts the functional architecture of grid cells. eLife, 4, e08362.
1. Wei, X. X., & Stocker, A. A. (2012). Efficient coding provides a direct link between prior and likelihood in perceptual Bayesian inference. In Advances in neural information processing systems (NeurIPS).